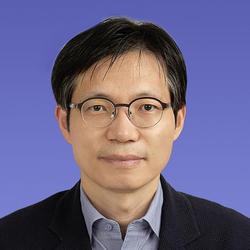
Classification of white blood cells by deep learning
A Talk by Prof. Jon-Lark Kim (Sogang University, Seoul, Korea)
About this Talk
The classification of white blood cells has been one of the important problems in the area of medical image processing and AI data analysis. Most current research on this is based on the established datasets including BCCD, LISC, and segmentation WBC. The algorithms known so far have not been tested for other datasets. Hence, it is a natural question to develop a more general deep learning algorithm which works for various kinds of datasets of WBC.
In order to be more realistic, we collected white blood cell images from actual patients and integrated them with the known datasets. We have developed a CNN model called DH-WBC classifier with 10 layers which outperforms VGG-16 and ResNet-50 with respect to the classification accuracy. Further, we have applied YOLO for object detection and structure-preserving color normalization and U-net for image segmentation.
Our DH-WBC classifier accurately identifies five types of white blood cells, with the achievement of over 97% classification accuracy using a dataset of over 30,000 images.
We have proposed a full deep learning network whose input is any blood cell image and whose out is one of the five WBCs. Our accuracy is one of the best results in this area.